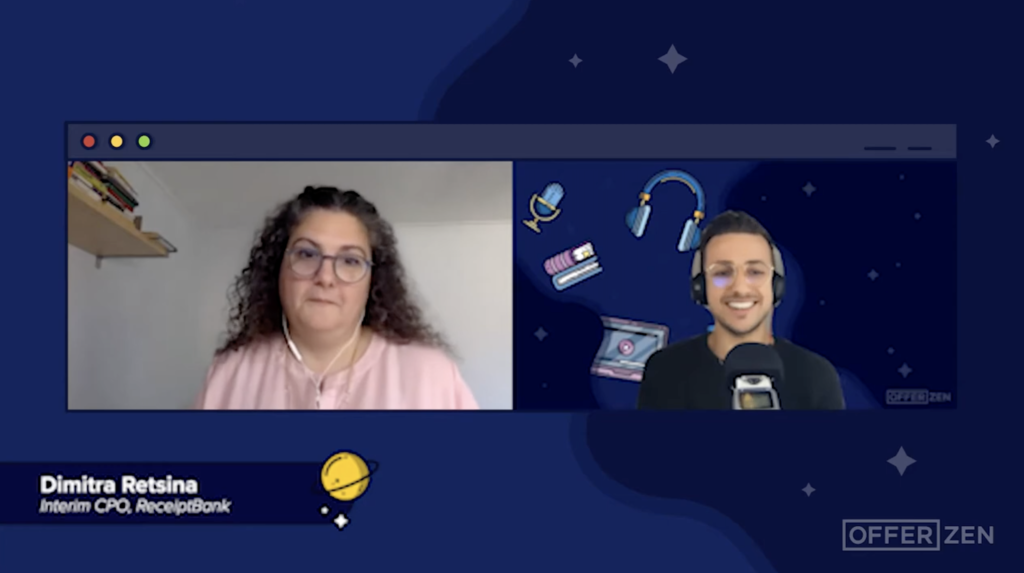
Data-informed Product Strategy is not a new topic. Although I discussed this topic at OfferZen’s podcast in the midst of the pandemic, the points touched continue to be relevant to any product leader today. This discussion took place at the time I served as CPO (a.i.) at Dext (as ReceiptBank was rebranded), UK accounting platform operating globally. Before joining Dext (ReceiptBank), I was the VP of Product Operations at Bynder in the Netherlands, a DAM platform which also operated in multiple countries.
Below key takeaways from this podcast:
1. The Importance of Data in Product Strategy
Data is vital in confirming assumptions and guiding investments, especially for scale-ups where stakes are higher. It helps in making informed decisions that align with growth, EBITDA optimisation, and other business objectives.
2. Challenges for Product Leaders
Product Leaders always need to navigate uncertainty, address changing customer behaviours, retain customers and contribute to growth. It’s crucial to scrutinise investments, optimize digital acquisition through different channels, and sometimes explore M&A opportunities and altered market dynamics.
3. Product Operation Strategy
A data-led product strategy is essential. At Bynder, I focused on data-driven insights to inform product decisions. Similarly, at ReceiptBank, I emphasized understanding if the actions align with business goals as well as what the effectiveness of those actions were. Eventually we need to know if we are doing what we should be doing. A data-led strategy validates gut feelings and measures ROI, providing crucial insights for scale-ups. It combines various data sources, offering a comprehensive view for better decision-making, aligning financial performance with product efforts, and ensuring effective strategic investments.
For example, at Bynder, we prioritized roadmap initiatives based on desired business impact, such as retention, growth, or cost optimization. By analyzing the ROI of different initiatives, we could make data-driven decisions that maximized investment impact.
4. Type of Data to be advised from
Product Leaders need to be looking at data such as financial metrics like ARR, but also CRM system data, support requests, and usage behavior insights. Combining these data types helps prioritize initiatives based on business impact, ensuring strategic alignment and effective resource allocation. Data-driven insights allow for informed discussions. For example, a product manager at ReceiptBank shifted priorities after analyzing business case data, leading to healthier decision-making processes within the product organization.
5. How to Start Using Data
Begin by identifying where data exists, ensuring it’s clean and accessible. Collaborate with finance, support, and other departments to gather comprehensive insights. Start small but ensure accuracy, and progressively combine data from different systems. Start by combining basic systems like finance and CRM. Gradually expand data integration, focusing on clean, reliable data to support sound decision-making. The most significant lesson is to start integrating data and continuously question its accuracy. Ensuring reliable data and validating insights is crucial for effective product strategy.
6. Where to Find Data
Identify the business goal you’re addressing, then partner with other departments to locate relevant data. Combining data from finance, CRM, and support systems with product data provides a holistic view, enabling better strategic decisions. Focus on key insights like product financials, engineering investments, and user behavior. Understanding ARR, cost structures, and customer segmentation helps prioritize initiatives that align with business goals.
7. Investments Context is Important
Categorizing engineering investments—whether in technical debt, sales debt, or growth—provides a health check. It ensures alignment between product goals and actual resource allocation, helping identify and address discrepancies. Analyze resource allocation to understand investment distribution. Unexpected investments, like excessive custom work, indicate potential issues with scalability or product-market fit, prompting strategic adjustments.
8. Data Tools and Visualizing Insights
Combining data in a central repository, like a data lake, and using BI tools to create insights and views is effective. This integration facilitates comprehensive data analysis and informed decision-making. You can track user behavior with tools like Amplitude, Mixpanel or Pendo, integrated via data hubs like Segment. Understanding user journeys and behaviours helps refine product features and improve user experiences. Combining finance, sales, and user behavior data provides a multi-faceted view. For example, analyzing cost optimization alongside user behavior ensures investments align with strategic goals, enhancing decision-making.
For example, at Bynder, analyzing product add-ons profitability revealed underused features. Partnering with customer support, we managed discontinuation cycles, minimizing customer disruption and optimizing resource allocation.
Link to the podcast “How to Build Data-Driven Product Roadmaps, Dimitra Retsina CPO (a.i.) ReceiptBank”